If you wanted to know the average weight of zebra mussels in Lake Superior, the only way to know for certain would be to capture every single one, count them and weigh them individually. Obviously this is impractical.
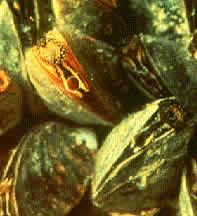
Even if you had the money for such a crazy scheme, the mussels would probably reproduce faster than you could weigh them. The answer is to weigh a relatively small number of mussels, and use the average of this smaller collection as an approximation of the true average. This is what sampling is all about.
There are millions of zebra mussels in Lake Superior, but we can learn about this large population, by looking at a small but representative sample. We start out wanting to know some characteristic about a population. The population is everything we’re interested in knowing about. Populations can be well defined, for example mussels in a specific lake; somewhat defined, such as grains of sand on Pacific Northwest beaches; or completely hypothetical, as for example all rats reared at 25 C and fed a diet of Purina Rat Chow. Properties which characterize populations are called parameters. Examples for a zebra mussel population would include average weight, most common colour, or how much shell length varies from individual to individual.
Since we typically can’t measure all individuals in our population of interest, we take a smaller sample and estimate our parameter from that. Our estimate is called a statistic.
Obviously we want our statistic to be as close as possible to the true parameter value. How can we accomplish this?
Bigger Sample:
One obvious way is to take a bigger sample. It should be obvious that as our sample size approaches the size of the population, our sample statistic will approach the true parameter. Where practical, a bigger sample is always better, but keep in mind that there are diminishing returns for a larger and larger sample size. See also Replication below.
Unbiased Sample:
Our sample should be unbiased, meaning that every part of our population should have an equal chance of being included in the sample. If bigger mussels are more likely to be collected, our sample average will be larger than the true average. If small mussels get collected more often, the reverse will be true. Biases can come from many directions including:
- Ease of sampling (we may prefer to collect our mussels where the water is shallower).
- Perceptions (larger mussels may be more visible).
- Equipment (small mussels might slip through a net, or large mussels might cling more tightly to rocks).
- Unconscious preconceptions (we might expect the average mussel to be small and so unconsciously tend to select smaller ones).
Random Sample:
The simplest, and usually best, way to get an unbiased sample is to randomly choose your sample organism or sample site. For example with our zebra mussels we might divide the lake up into a grid of 1000 locations, give each a number, and then randomly draw numbers from a hat to decide which locations are sampled.
Transect:
Another technique that is sometimes used to get a representative sample is the transect. If we know that there is a gradient within our population, we can draw a line following the gradient, and sample along it. For example, suppose we are estimating the density of woodpecker nests in a forest, and we know that nests are more common in the western edge of the forest where trees are thicker. We would draw our transect from west to east to ensure we sampled both in areas where nests are common and where they are rare. Using a transect does not mean giving up all randomness. The location of the transect (between the northern and southern edges of the forest) could be chosen randomly, and sampling sites picked at random distances along the transect.
Replication:
It’s probably obvious to you that if you want to know something about a population you will need to collect more than one data point. Almost everything biologists are interested in is variable. Whether we’re measuring DNA sequences in microbes, hormone levels in plants, or behavior in animals, we have to cope with the fact that every individual is a little (or a lot!) different. We want to see the broad patterns that apply to whole groups of organisms, so we need to have a large enough sample to see past the random variations or “noise.”
Within Group and Between Group Variation:
More precisely, collecting a larger sample helps us to distinguish variation within a group (the noise) from variation between groups (usually what we are interested in). Let’s say we want to know whether Cushion Milk Vetch (Astragalus gilviflorus) has seed pods which are smaller than its close relative Prickly Milk Vetch (Astragalus kentrophyta). If we measured only a single seed pod from each species, we would certainly find a difference, but we would have no way of knowing whether the difference was due to random variation within each species, or a “real” difference between species. But if we measure at least a few plants in each species, we get a better estimate of how much variation exists within each species, and also a better estimate of average pod lengths for each species. We can then use inferential statistics to estimate how likely it is that the averages are really the same (remember we try to disprove our null hypothesis). The more individuals we test, the more we will know about what is going on within each species, and the more power we will have to detect differences between species.
How Big a Sample?
Bigger samples are always better, but not always enough better to justify the time and expense because there are diminishing returns with larger and larger sample size. Increasing your sample size from 10 to 20 will have a much bigger effect on reliability of the statistics than increasing from 20 to 30, and increasing from 30 to 40 will make even less of an improvement. There is no universal “best” sample size. If your population is highly variable, you will need a larger sample to get a good estimate; if your population is quite uniform, a small sample will be enough.
Pseudoreplication:
Returning to the example of the Milk Vetches, it’s easy to see that it would not be proper to simply measure the same seed pods over and over again to increase sample size. Measuring the same pod over and over again doesn’t tell us anything new about variation between seeds. Similarly, taking all our pods from a single plant would not be a good idea either because pods from the same plant might be more similar than pods from other plants. They would not be independent, and we would be guilty of pseudoreplication (pseudo means false or phony) rather than true replication. If we want our sample to be representative of all plants, then all plants should have an equal probability of being sampled. Getting totally independent samples may be a challenge. Just as pods from a single plant may not be representative of all Milk Vetch in the Lethbridge coulees, plants in the Lethbridge coulees may not be representative of all Milk Vetch everywhere. The best solution is to sample as widely as possible, but time and budget constraints can put limits on this, especially for student projects. When you are drawing conclusions from the results of your experiment (in the Discussion section of your paper) consider what population you have really sampled, and limit your conclusions to that population. If you only sampled Astragalus in the the Lethbridge coulees, be careful about extending your conclusions to all Astragalus everywhere.